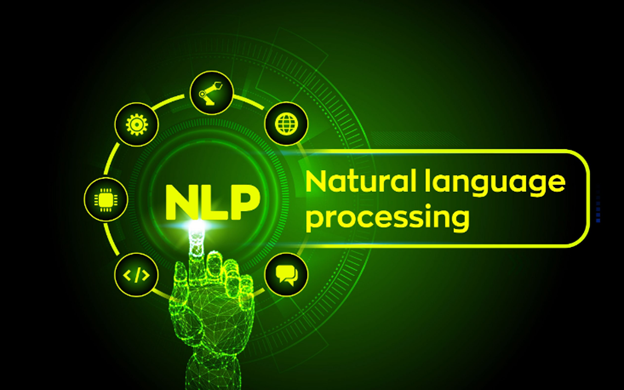
What is natural language processing?
Natural language processing (NLP) is the ability of a computer program to understand human language as it is spoken and written — referred to as natural language. It is a component of artificial intelligence (AI).
NLP has existed for more than 50 years and has roots in the field of linguistics. It has a variety of real-world applications in a number of fields, including medical research, search engines and business intelligence.
How does natural language processing work?
NLP enables computers to understand natural language as humans do. Whether the language is spoken or written, natural language processing uses artificial intelligence to take real-world input, process it, and make sense of it in a way a computer can understand. Just as humans have different sensors — such as ears to hear and eyes to see — computers have programs to read and microphones to collect audio. And just as humans have a brain to process that input, computers have a program to process their respective inputs. At some point in processing, the input is converted to code that the computer can understand.
There are two main phases to natural language processing: data preprocessing and algorithm development. Data preprocessing involves preparing and “cleaning” text data for machines to be able to analyze it. preprocessing puts data in workable form and highlights features in the text that an algorithm can work with. There are several ways this can be done, including:Tokenization :
This is when text is broken down into smaller units to work with.Stop word removal :
This is when common words are removed from text so unique words that offer the most information about the text remain.
Lemmatization and stemming :
This is when words are reduced to their root forms to process.Part-of-speech tagging :
This is when words are marked based on the part-of speech they are — such as nouns, verbs and adjectives.
Once the data has been preprocessed, an algorithm is developed to process it. There are many different natural language processing algorithms, but two main types are commonly used:
Rules-based system :
This system uses carefully designed linguistic rules. This approach was used early on in the development of natural language processing, and is still used.
Machine learning-based system :
Machine learning algorithms use statistical methods. They learn to perform tasks based on training data they are fed, and adjust their methods as more data is processed. Using a combination of machine learning, deep learning and neural networks, natural language processing algorithms hone their own rules through repeated processing and learning.
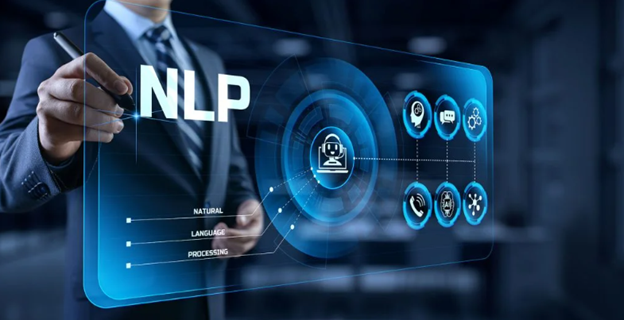
What is natural language processing used for?
Some of the main functions that natural language processing algorithms perform are:- Text classification
- Text extraction
- Machine translation
- Natural language generation
The functions listed above are used in a variety of real-world applications, including:
- Customer feedback analysis : where AI analyzes social media reviews.
- Customer service automation : where voice assistants on the other end of a customer service phone line are able to use speech recognition to understand what the customer is saying, so that it can direct the call correctly.
- Automatic translation : using tools such as Google Translate, Bing Translator and Translate Me.
- Academic research and analysis : where AI is able to analyze huge amounts of academic material and research papers not just based on the metadata of the text, but the text itself.
- Analysis and categorization of medical records : where AI uses insights to predict, and ideally prevent, disease.
- Word processors used for plagiarism and proofreading : using tools such as Grammarly and Microsoft Word.
- Stock forecasting and insights into financial trading : using AI to analyze market history and 10-K documents, which contain comprehensive summaries about a company’s financial performance.
- Talent recruitment in human resources and,
- Automation of routine litigation tasks : one example is the artificially intelligent attorney.
Benefits of natural language processing
- Improved accuracy and efficiency of documentation.
- Ability to automatically make a readable summary of a larger, more complex original text.
- Useful for personal assistants such as Alexa, by enabling it to understand spoken word.
- Enables an organization to use chatbots for customer support.
- Easier to perform sentiment analysis and
- Provides advanced insights from analytics that were previously unreachable due to data volume.
Challenges of natural language processing
- Precision
- Tone of voice and inflection
- Evolving use of language
The evolution of natural language processing
NLP draws from a variety of disciplines, including computer science and computational linguistics developments dating back to the mid-20th century. Its evolution included the following major milestones:
- 1950s : Natural language processing has its roots in this decade, when Alan Turing developed the Turing Test to determine whether or not a computer is truly intelligent. The test involves automated interpretation and the generation of natural language as criterion of intelligence.
- 1950s-1990s : NLP was largely rules-based, using handcrafted rules developed by linguists to determine how computers would process language.
- 1990s : The top-down, language-first approach to natural language processing was replaced with a more statistical approach, because advancements in computing made this a more efficient way of developing NLP technology. Computers were becoming faster and could be used to develop rules based on linguistic statistics without a linguist creating all of the rules. Data-driven natural language processing became mainstream during this decade. Natural language processing shifted from a linguist-based approach to an engineer-based approach, drawing on a wider variety of scientific disciplines instead of delving into linguistics.
- 2000-2020s : Natural language processing saw dramatic growth in popularity as a term. With advances in computing power, natural language processing has also gained numerous real-world applications. Today, approaches to NLP involve a combination of classical linguistics and statistical methods.
Techniques and methods of natural language processing
- Parsing
- Word segmentation
- Sentence breaking
- Morphological segmentation
- Stemming
- Word sense disambiguation
- Named entity recognition
- Natural language generation